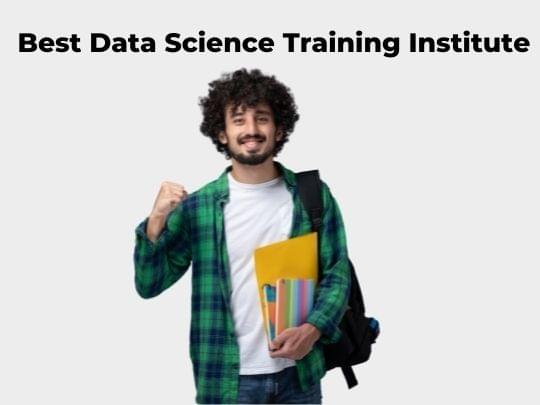
It is coupled along with the actionable insights discovered via the method of the Data Science Life Cycle. The first thing to be carried out is to collect data from the information sources obtainable. There are special packages to read knowledge from specific sources, corresponding to R or Python, proper into the information science programs. You might discover quite a few kinds of databases, similar to Oracle, PostgreSQL, and MongoDB. Yet another difference is to acquire data by way of Web APIs and crawling knowledge. Social media websites similar to Twitter and Facebook let their users approach knowledge by connecting with web servers. This step involves getting some idea about the resolution and elements affecting it earlier than constructing the precise mannequin.
Hence this work is usually accomplished by data analysts and typically by data scientists. If you enjoy content like this on all things information science, Machine Learning, AI, Web, and Mobile App Development, visit our facebook Page and youtube channel for extra content. On high of that, you will want to visualize your findings accordingly, maintaining it driven by your small business questions. It is very important to find a way to current your findings in such a method that's useful to your organization, or else it would be pointless to your stakeholders. In short, regression and predictions are used to forecast future values, and classification is so that you simply can determine and group your values. Lastly, in this step, you can also practice fashions to carry out classification.
Actionable insights from the model reveal how Data Science has the ability to do predictive analytics and prescriptive analytics. This gives us the power to discover ways to repeat optimistic outcomes or the means to prevent the negative end result. After the modeling process, model efficiency measurement is required. For this precision, recall, F1-score for classification drawback might be used. If it is overfitted mannequin then predictions for future information won't come out precisely. It is important to establish what's the ask, is it a classification problem, regression or prediction problem, time series forecasting, or a clustering downside.
Click here to know more about Data Science Institute in Bangalore
Data Science is an area that rewards you nearly higher than some other area, however, asks you to comply with a certain professional path to be a deserving information scientist. First of all, you need to acquire a bachelor’s diploma in Computer Science, Information Technology, or Mathematics. After finishing your degree, you need to get an entry-level job as a data analyst or a junior data scientist for expertise before moving into the massive games. You can get your master’s parallelly along with your entry-level job too. After finishing your greater studies, you possibly can apply for the publish of a senior data scientist.
Therefore, upon getting received your corporation hypothesis prepared, it is sensible to spend so much effort and time here. With that, we hope you could have gained a better understanding of how an information science project is carried out from finish to finish. If you’re ready to ensure the information initiatives you work on always stay on track and profitable, let’s dive into the information science course of lifecycle framework. Data collection entails acquiring data from each known inside and external source that can help in addressing the enterprise concern.
Government businesses and public policy organizations are additionally huge customers. From an operational standpoint, data science initiatives can optimize management of provide chains, product inventories, distribution networks, and customer service.
Well, we can strategize all day lengthy - however, one major gap I’ve witnessed that I imagine could significantly be contributing to poor ROI is the lack of strategic and business information given to knowledge workers. And with the current trade information project failure rate sitting at 80%, it’s no surprise that knowledge professionals are careworn. After gaining clarity on the problem assertion, we need to gather relevant data to break the issue into small components. The globally accepted construction in resolving any kind of analytical drawback is popularly generally recognized as Cross Industry Standard Process for Data Mining or abbreviated as CRISP-DM framework. Business Understanding performs a key function in the success of any project. We have all the technology to make our lives simple but still with this large change a hit of any project is determined by the standard of questions asked for the dataset. Another popular possibility to gather data is connecting to Web APIs.
Too many corporations collect incomplete, unreliable data, and everything they do after that… is just messed up. So as I all the time say, step one is the toughest and most essential within the process so doing it correctly is extraordinarily essential. Other than classification or prediction of the outcomes, our objective of this stage can even embody the grouping of information to understand the logic behind those clusters. For instance, you would like to group your e-commerce customers to understand their behavior on your website. So this may require you to determine groups of knowledge points with clustering algorithms, utilizing strategies like k-means; or make predictions using regressions like linear or logistic regressions.
Data acquired in the first step of an information science project is normally not in a usable format to run the required analysis and would possibly contain lacking entries, inconsistencies, and semantic errors. Additionally, this stage is known as Data Cleaning or Data Wrangling. By using feature engineering, you'll find a way to create new information and extract new features from present ones. Format the data in accordance with the specified construction and delete any pointless columns or capabilities.
Data science entails analytics purposes that are more superior. In order to acquire the correct data, we should always be in a position to understand the business. Asking questions about the dataset will help in narrowing right down to the right data acquisition. In any case, once we've modeled the information we will derive insights from it. This is the stage where we can finally start evaluating our complete information science system.
Visit to know more about Data Science Course in Bangalore
Navigate to:
360DigiTMG - Data Science, Data Scientist Course Training in Bangalore
No 23, 2nd Floor, 9th Main Rd, 22nd Cross Rd, 7th Sector, HSR Layout, Bengaluru, Karnataka 560102
1800212654321
Get Direction: Data Science Institute