Abilities of Data Science Courses in Bangalore
Abilities of Data Science Courses in Bangalore
Data Science
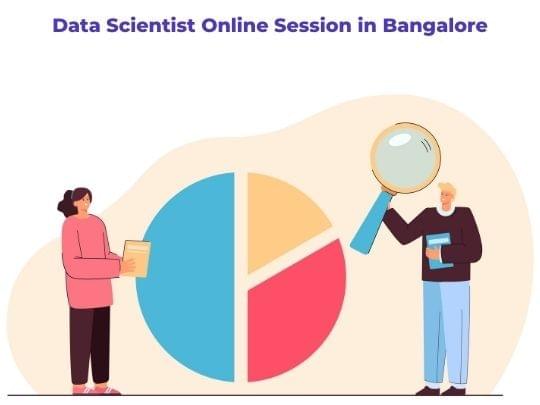
Huge Data And Discrimination
Here, the primary focus ought to be on evaluating each societal notions of “fairness” and attainable social costs. This impact is known as “algorithmic bias” and is becoming a standard issue for information scientists. Google didn’t go out of its way to be racist, Facebook didn’t intend to get customers arrested and IBM et al didn’t determine to make their facial recognition software blind to black ladies.
These examples spotlight the importance of excited about how biases would possibly impression the study of educational information and how data-driven fashions used in instructional context may perpetuate inequalities. To perceive this question, we ask whether or not and how demographic information, together with age, educational-level, gender, race/ethnicity, socioeconomic standing and geographical location, is used in Educational Data Mining analysis. This survey exhibits that, though a majority of publication reported at least one category of demographic info, the frequency of reporting for different categories of demographic data may be very uneven (ranging from 5% to 59%) and only 15% of publications used demographic information of their analyses. Recently, much consideration has been paid to the societal influence of AI, especially considerations concerning its equity. A growing physique of research has recognized unfair AI systems and proposed methods to debias them, but many challenges stay. Representation studying for Heterogeneous Information Networks , a elementary building block used in advanced network mining, has socially consequential purposes similar to automated profession counseling, however there have been few attempts to ensure that it will not encode or amplify dangerous biases, e.g. sexism within the job market. To address this gap, in this paper we suggest a complete set of de-biasing methods for honest HINs illustration learning, including sampling-based, projection-based, and graph neural networks -based strategies.
Scholars are somewhat sceptical about finding an answer to this drawback as a end result of ever-changing technological panorama that creates new inclusion difficulties . Still, due to the potential promising helpful purposes of Big Data technologies, extra studies ought to give attention to the analysis and implementation of such truthful makes use of of data-mining while contemplating and avoiding the creation of recent divides. In most circumstances, information scientists deliberately design algorithms to be blind to protected classes similar to race, religion and gender. They implement this safeguard by prohibiting predictive fashions — that are the formulation that render momentous choices corresponding to pretrial launch determinations — from contemplating such elements. Unbiased knowledge collection is important to guaranteeing fairness in synthetic intelligence fashions.
They had been ‘victim’ of the setting their AI realized from and the negative impression on people’s lives was collateral injury of this limitation. Second, without an enough definition of discrimination, it's difficult for laptop scientists and programmers to appropriately implement algorithms. In fact, to keep away from unfair practices, measure equity and quantify unlawful discrimination , they need to translate the notion of discrimination into a formal statistical set of operations. The want for this expert knowledge may clarify why, compared to other researchers within the subject, laptop scientists have been at the forefront of the seek for a viable definition. Although the majority of papers have been theoretical in nature, the time period discrimination was introduced as self-explanatory and linked to other notions such as injustice, inequality and unequal remedy, excluding some papers in legislation and laptop science. This overall lack of a working definition within the literature is highly problematic, for several causes.
Click here for more information on Best Data Science Courses in Bangalore
In this paper, we bring together the analysis of ethical dilemmas in schooling and the necessity to incorporate moral reasoning into the AI systems’ decision procedures. Without due precautions, machine learning’s choices meet the very definition of inequality. For instance, for informing pretrial launch, parole and sentencing choices, the model calculates the probability of future felony convictions. If the info hyperlinks race to convictions — displaying that black defendants have greater than white defendants — then the resulting model will penalize the score for every black defendant, just for being black, unless race has been deliberately excluded from the model.
Doing so will improve algorithmic efficiency on the expanded set of aforementioned evaluation criteria, reducing disparities in care and in outcomes. The use of predictive tools similar to Northpointe's Correctional Offender Management Profiling for Alternative Sanctions software in felony justice to tell sentencing and parole decisions by predicting individual's' danger of reoffending offers another cautionary instance. An analysis of COMPAS predictions and subsequent rearrests in Broward County, Florida, by the NGO ProPublica concluded that COMPAS was biased against African American defendants, though Northpointe has disputed these conclusions.
In addition, the info set used to coach the predictive model must be clearly described, in order that the representativeness of the pattern inhabitants and any systematic biases which may impact the model predictions can be assessed. In the health care setting, these challenges, if not adequately addressed, may impede health fairness. For example, an algorithm trained on data from a nonrepresentative affected person inhabitants may fail to offer sufficient predictions in different settings. Even if the data are representative, failure to account for heterogeneity inside the affected person inhabitants could result in suboptimal predictions for patients with unusual variants of a illness. Target variable bias may manifest if we fail to account for whether or not patients will adjust to a given therapy regimen, measuring the benefits to adherent patients only. Finally, as we extra exactly predict the dangers and advantages of remedy for various circumstances, there's a danger that we'll preferentially direct restricted well being care sources to these subpopulations with the best cost/benefit trade-offs. This could result in systematic biases in well being look after minority groups—who may respond in a different way to treatments developed for the majority.
If the info used to coach the algorithm are extra consultant of some groups of individuals than others, the predictions from the mannequin may also be systematically worse for unrepresented or under-representative groups. For example, in Buolamwini’s facial-analysis experiments, the poor recognition of darker-skinned faces was largely because of their statistical under-representation in the training knowledge. That is, the algorithm presumably picked up on sure facial options, corresponding to the space between the eyes, the form of the eyebrows and variations in facial skin shades, as ways to detect male and female faces. However, the facial features that have been extra representative in the coaching knowledge were not as numerous and, subsequently, less reliable to tell apart between complexions, even leading to a misidentification of darker-skinned females as males.
Thus, finding out employees' ability to steadiness reliance on algorithmic recommendations and significant judgment in direction of them, holds immense significance and potential social acquire. In this study, we targeted on gig-economy platform employees and easy perceptual judgment tasks, by which algorithmic errors are relatively visible. In a collection of experiments, we present staff with misleading recommendation perceived to be the results of AI calculations and measure their conformity to the faulty suggestions. Our initial results point out that such algorithmic recommendations hold strong persuasive energy, even compared to suggestions which are presented as crowd-based. Our research additionally explores the effectiveness of mechanisms for lowering workers' conformity in these situations. That’s why sometimes, even when the information suggests in any other case, it’s a good idea to let folks make the final judgment call. For instance, when Amazon determined to roll out same-day supply in New York, their system determined predominantly white neighborhoods had been the best locations to begin.
Click here for more information on Data Science Certification in Bangalore
Navigate To:
360DigiTMG - Data Science, Data Scientist Course Training in Bangalore
Phone: 1800-212-654321
Read more Blogs
Read more Articles