Data Science Course Bangalore
Data Science
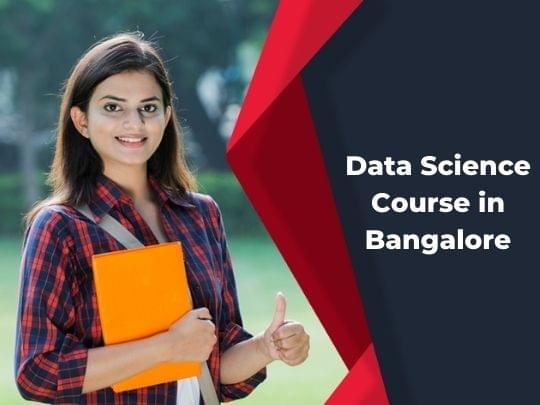
Data Engineer Interview Questions and Answers
This schema is used for querying massive data sets. One might illustrate under the excessive-stage architecture of the data model.
For dealing with unstructured knowledge, R supplies an enormous variety of support packages. Python is greatest apt at handling colossal information whereas R has memory constraints and is slower in response to giant knowledge. Therefore, the choice for utilizing Python or R depends on the area of functionality and usage. In order to train this algorithm, we require labeled data. K-means is an unsupervised studying algorithm that looks for patterns that are intrinsic to the info. The Kin KNN is the number of nearest data factors.
In HDFS, the balancer is a managerial utilized by admin workers to rebalance information throughout DataNode and strikes blocks from over-utilized to underutilized nodes. A replication issue is a whole number of replicas of a file in the system.
The query is asked to evaluate your previous expertise within the field. The interviewer right here desires to know which steps or precautions you will take during information preparation. Begin by explaining that information preparation is required to get necessary data which can then further be used for modeling functions. Emphasize the type of mannequin you will use and your reasoning behind the selection. The primary role of the JobTracker is resource administration, which basically means managing the TaskTrackers. Apart from this, JobTracker also tracks useful resource availability and handles task life cycle administration. Data Ingestion – This is the first step within the deployment of a Big Data solution.
Click here to know more about Data Science Course in Bangalore
It’s faster to query unstructured data from a NoSQL database than it's to query JSON fields from a JSON-type column in PostgreSQL. You can at all times do a pace compatibility check for a definitive answer. A relational database is one where data is saved in the form of a desk. Each table has a schema, which is the columns and kinds a document is required to have.
It happens when there isn't any information worth for a variable in an observation. If lacking values usually are not handled properly, it's sure to result in faulty information which in turn will generate incorrect outcomes. Thus, it is extremely beneficial to deal with lacking values appropriately earlier than processing the datasets. Usually, if the number of missing values is small, the information is dropped, but if there’s a bulk of missing values, information imputation is the preferred plan of action.
Each schema will need to have a minimum of one primary key that uniquely identifies that document. In other words, there are not any duplicate rows in your database. Moreover, each desk can be related to other tables utilizing overseas keys. “A profitable information engineer needs to know tips on how to architect distributed techniques and information shops, create dependable pipelines and combine data sources successfully. Data engineers additionally want to have the ability to collaborate with staff members and colleagues from different departments. As a knowledge engineer, I will most probably have a better perspective or understanding of the information throughout the company.
The embedded methodology combines the best of both worlds – it consists of one of the best options of the filters and wrappers methods. In this technique, the variable selection is done in the course of the coaching process, thereby allowing you to establish the features which are the most correct for a given mannequin.
The primary aim of feature selection is to simplify ML models to make their evaluation and interpretation easier. Feature choice enhances the generalization abilities of a mannequin and eliminates the problems of dimensionality, thereby, preventing the possibilities of overfitting. Feature choice refers to the strategy of extracting only the required options from a selected dataset. When data is extracted from disparate sources, not all knowledge is helpful always – completely different business needs names for various data insights. This is where characteristic selection is available to identify and select only these features that are related to a selected business requirement or stage of knowledge processing. Overfitting refers to a modeling error that occurs when an operation is tightly matched by a limited set of knowledge factors.
When it comes to this algorithm, Map and Reduce kind of operations are the ones that are used for processing the big information units. The Map technique is the one that does the filtering and sorting of the actual data the Reduce methodology also performs summaries of the information.
Visit to know more about Data Science Institute in Bangalore
Navigate to:
360DigiTMG - Data Science, Data Scientist Course Training in Bangalore
No 23, 2nd Floor, 9th Main Rd, 22nd Cross Rd, 7th Sector, HSR Layout, Bengaluru, Karnataka 560102
1800212654321
Visit on map: Data Science Course