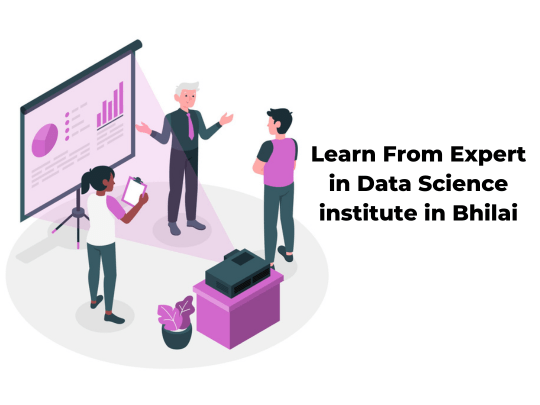
Statistics is a fundamental and crucial component in the toolkit of a full-stack data scientist. Here are several reasons why statistics is important in this role:
Data Exploration and Understanding:
Statistics allows data scientists to explore and understand the underlying patterns and characteristics of the data they are working with. Descriptive statistics provide insights into central tendencies, variations, and distributions.
Informed Decision-Making:
Statistical methods help in making informed decisions based on data. Whether it's choosing an appropriate model, evaluating its performance, or drawing conclusions from experiments, statistical techniques play a vital role.
Hypothesis Testing:
Statistics is crucial for hypothesis testing, which is used to validate assumptions and draw conclusions about populations based on samples. This is important in both traditional statistical analysis and in machine learning.
Machine Learning Model Evaluation:
In machine learning, statistical metrics are used to evaluate the performance of models. Concepts such as precision, recall, F1 score, and ROC-AUC are all rooted in statistical principles.
Sampling Techniques:
Understanding statistical sampling methods is essential for collecting representative data. This is particularly important when dealing with large datasets or making predictions based on samples. Check ouu the Data Science Course in Bhilai
Probability:
Probability concepts are fundamental in understanding uncertainty and randomness in data. Many machine learning algorithms, particularly those based on probabilistic models, rely on a solid understanding of probability.
Statistical Inference:
Statistical inference is the process of drawing conclusions about populations based on sample data. This is a key aspect when making predictions or generalizing findings from a dataset to a larger context.
Experimental Design:
When conducting experiments or A/B testing, statistical methods help design experiments, analyze results, and draw valid conclusions.
Dealing with Variability:
Statistics provides tools to quantify and manage variability in data, which is essential for understanding the reliability of results and predictions.
Bayesian Methods:
Bayesian statistics is increasingly used in data science, especially for handling uncertainty and updating beliefs based on new evidence.
In summary, statistics is foundational for a full-stack data scientist because it provides the tools and techniques to make sense of data, draw meaningful insights, and make informed decisions in both traditional statistical analysis and modern machine learning applications.
Kickstart your career by enrolling in this Best Online Data Science institute in Bhilai
Navigate To:
360DigiTMG - Door No: 244, Zonal Market,Sector 10, Bhilai, Dist-Durg,
Chhattisgarh - 490006
Email: bhilai@360digitmg.com
Phone:+91 98866 28363/ +91 99816 17903